Notebook Activities
Contents
Notebook Activities#
Notebooks offer a clean interface to provide lesson content, visualizations, and instructions for proceeding through an LX.
At the core, a Duckietown Learning Experience notebook has all the possibility of a classic Jupyter notebook but with every library and functionality from the Duckietown ecosystem added in.
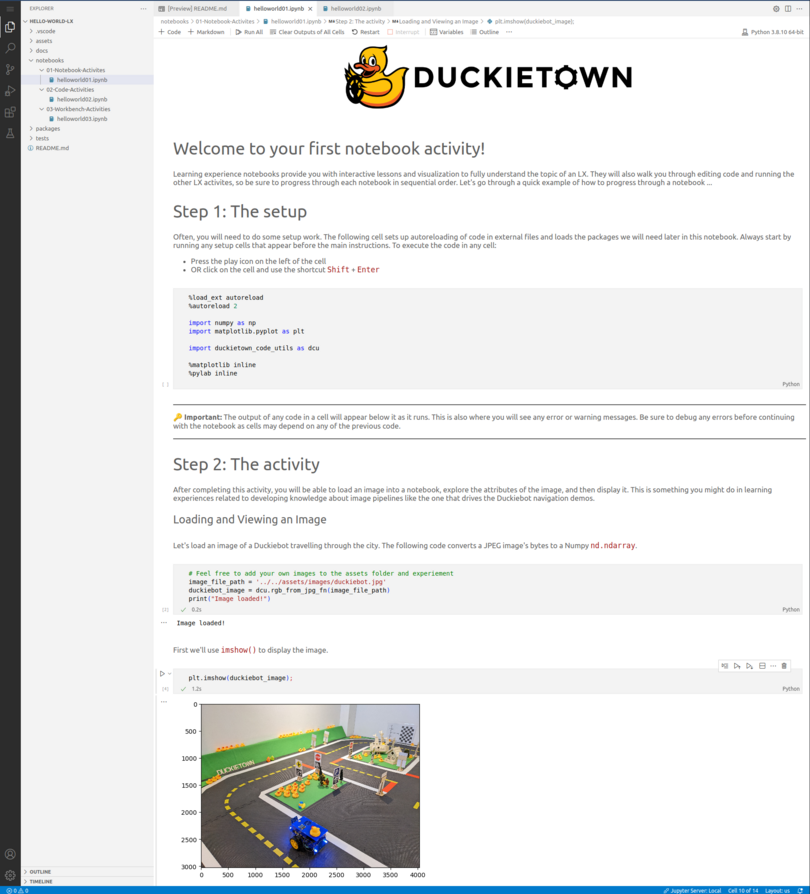
Fig. 13 Each challenge and linked learner submissions can be found on the Duckietown Challenges Server.#
Take a moment to explore the notebooks in the Demo Learning Experience to see a few of the integration options.
Edit zones#
The following table contains a list of the files and directories that you may need to update to implement this type of LX activity. If you would like a full walkthrough showing how to implement notebooks, skip to the next section.
Feature |
File Location |
Purpose |
---|---|---|
Jupyter Notebook |
|
Notebook files contain the knowledge portion of an LX, walking students through activities, visualizations, and development. |
Learner Solution Code |
|
Python files for learner implementations should remain in the |
Notebook Dependencies |
|
Required libraries are built into the VSCode editor environment by including them in the |
Development Guidelines#
Notebooks vs packages:
Notebooks vs. Packages: As a general rule, example code and visualizations should be developed by students directly in the notebooks.
Code for
agents and other packages should be placed in the packages/solutions
directory for students to edit in the
respective Python files, then imported into the notebooks and unit tests for visualization and testing of their results.
A note on solutions:
Notebooks should generally walk through the knowledge required to implement some behavior on the Duckiebots, providing solutions to every notebook activity along way until the culminating activity in the final notebook.
Students should then be editing package files to implement their work as directed by the notebook instructions, and the solution should be hidden in the separate solutions repository to enable evaluation.
Notebook length:
The format of a Duckietown Learning Experience paired with the dts code
workflow enables students to iteratively edit and run solutions as they walk through notebookds and develop their understanding of a topic.
Within this framework, shorter notebooks with concrete goals that build learner skills up to a final agent challenge are more effective than one long, content-heavy notebook.
Tutorial: Adding Content to Notebooks#
Step 0: Confirm your LX workspace setup#
The first step after creating a new development project should always be to run dts code build --recipe ../recipe
in the
<your-lx-workspace>/lx
directory to ensure that the template was initialized properly.
Step 1: Determine the learning and development goals#
Defining the learning goal will inform the content and visualization that you add to the notebook.
Defining a development goal will allow you to set learners up with a clear purpose and provide tests that ensure they successfully completed any coding exercises included in the notebook or a linked solution script.
Step 2: Determine dependencies#
If possible, determine the required Duckietown and external libraries and add them to a setup cell at the beginning of the notebook. This will confirm a lack of later import errors.
You can install external libraries by adding to the recipe/dependencies-apt.txt
and recipe/dependencies-py3.txt
files. Any dependencies added here will be available in the VSCode editor environment.
Step 3: Content and code recommendations#
Content is added to a Duckietown Learning Experience notebooks in the same Markdown format as any standard Jupyter notebook. For more information, see the Jupyter notebook docs.
As noted above, students should be editing package files to implement their work as directed by the notebook instructions, and the solution should be hidden in the separate solutions repository to enable evaluation.
This means that there are three places you can choose to have learners write solution code.
Directly in the notebook cells. This should be used for content examples and practice.
In a solution python script in the
lx/packages/solution
directory that is imported into the notebook for visualization. All functions should be predefined with clear TODOs marked for learners to complete as directed by the notebook.In a solution python script in the
lx/packages/solution
directory that is then imported into the Duckiebot agent code located in therecipe
for simulation and workbench activities (more on this in the following sections).
As a general rule, notebooks do not have access to the packages in the recipe/solutions
directory where the base code is placed for Duckiebot agents. This is to prevent learners from editing agent code to a confusing or unusable state.
In summary: Place visualization and content practice code in the notebook. Place learner solution code in linked python scripts housed in the solutions directory.
Hint
Strengthen learner test-driven development (TDD) habits by using the Testing interface in the VSCode
editor to provide unit tests for each function to be completed in an LX.
This will confirm that their solution performs as expected before any attempts to run it in simulation or
on your Duckiebot. Note that the beaker symbol to open the Testing interface may not appear in the sidebar
until after one of the Python files in the packages
directory has been opened.
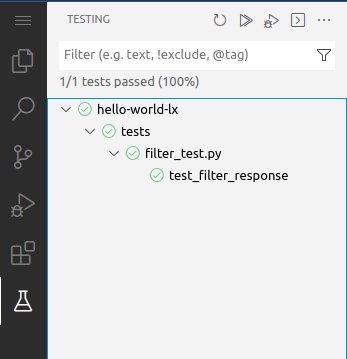
Step : Clean up and publish#
Clear all cells of output to avoid publishing solutions. Then follow the workflow in Publishing your LX to add your notebook and recipe updates to your Learning Experience repositories.